With the debut of ChatGPT, the AI technology has reached the most focused topic in the world. The general AI chips include CPU, GPU, and FPGA.
However, with the development of machine learning and edge computing, a large number of data processing tasks have put forward higher requirements for computing efficiency and computing energy consumption. Application Specific Integrated Circuits will be more distinctive in future AI development.
So, what is an ASIC? And will it replace the GPU in the future AI applications?
Introduction of Application Specific Integrated Circuits
An ASIC (Application Specific Integrated Circuits) is a chip that is custom-made for a specific electronic system.
In general, it is custom-designed based on the requirements of the specific application. Unlike the programmable logic device, ASIC is to achieve high performance for a custom task from the root level.
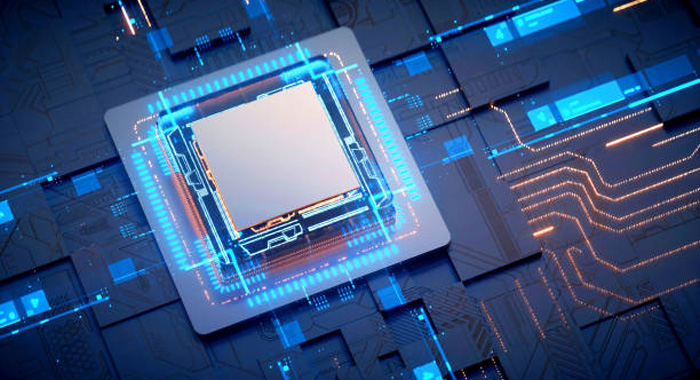
Source: Internet
During mass production, ASIC has the advantages of smaller size, lower power consumption, improved reliability, improved performance, enhanced confidentiality, and cost-effectiveness compared with general-purpose ICs.
It is performed greatly in a variety of applications. In data centers, ASIC accelerates tasks such as speeding up deep learning, artificial intelligence, and more. In communication equipment, it provides faster and more reliable data transmission. In aerospace and defense fields, it provides more efficient and precise control and monitoring solutions.
ASIC Compares with GPU, CPU, FPGA
As a general-purpose processor, in addition to meeting computing requirements, the CPU must be able to handle complex conditions and branches, as well as synchronization and coordination between tasks, to better respond to human-computer interaction applications. It requires a lot of space on the chip for branch prediction and optimization, and to cache to reduce the delay during task switching.
The GPU has a massively parallel computing architecture composed of thousands of smaller, more efficient ALU cores. It is better at handling multiple tasks, especially repetitive work without technical content, such as graphics calculations. Since deep learning usually requires a lot of training, which algorithm is not complicated but a large quantity of data. Compared with the CPU, GPU has the advantages of multi-core and parallel processing, so it is more suitable for deep learning operations.
FPGA (Field Programmable Gate Array) is also able to parallel process tasks. Uniquely, it is programmable. It means you can implement custom functions (Quick program according to the logic function requirement at any time).
ASIC is the best in individual aspects such as throughput, power consumption, and computing power level. The computing capacity and efficiency of ASIC chips are directly customized according to specific algorithm needs. Therefore, it can achieve more powerful performance and design requirements than the other three chips.
However, it will cost more in R&D, and take a long cycle of the initial R&D investment. Besides, because of the customization, it is barely replicable. It can not be easily repurposed or adapted for other tasks
Why is ASIC the Core of Future AI Development?
ASIC is not almighty, but it is the most necessary part of the coming AI field.
Remember the AlphaGo? Google released the first-gen TPU (Tensor Processing Unit) tensor processor in 2016. It is the ASIC chip that is designed for machine learning. And its most famous achievement is to use in training AlphaGo.
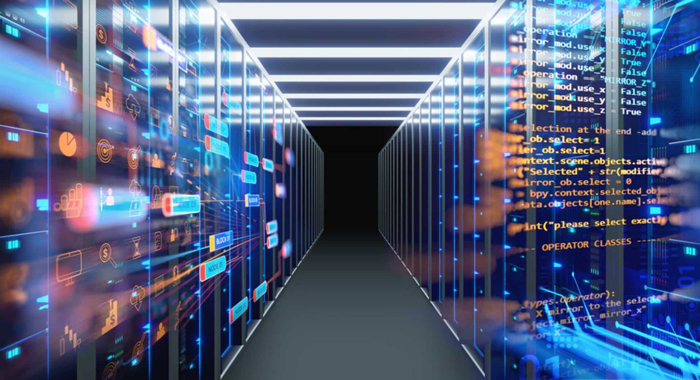
Source: Internet
According to Google, the processing speed of TPU is 15 to 30 times faster than GPUs or CPUs of the same period. And the energy efficiency is 30 to 80 times higher. Not only Google, but most manufacturers such as Intel, and Nvidia are also in developing.
With the development of science and technology, there are more and more application scenarios of AI technology, such as autonomous driving, face recognition, etc. The transfer of AI technology from the cloud to the terminal requires AI chips with stronger performance, efficiency, and smaller size. The GPU is not a chip specifically used for AI deep learning. When executing applications, its performance cannot be fully utilized, resulting in excessive energy consumption. So FPGA and ASIC began to become the protagonists.
FPGA is known as a ‘universal chip’. It can achieve almost all the functions you need. Significantly, it can be modified at any time, which is flexible.
However, in mass production, the cost of a single FPGA chip is higher than that of an ASIC chip. Its performance is also not as good as those of ASIC. That’s why manufacturers are focusing on ASIC in succession.
Artificial intelligence includes two parts: reasoning and training. The Training requires the chips to have a very high computing performance. The reasoning link has high requirements on simply specified repeated calculations and low latency.
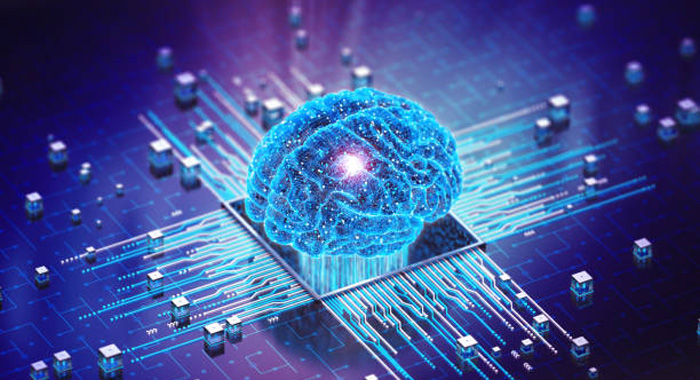
Source: Internet
The application scenarios of artificial intelligence can be divided into cloud and terminal. The training phase of deep learning requires a huge amount of data and a lot of calculations, and a single processor cannot complete it independently. So the training process can only be implemented on a cloud server. However, the amount of terminal data is huge and the demands vary greatly. So the inference link cannot be completed in the cloud. This requires various electronic units, hardware computing platforms, or domain controllers on the device to have independent inference computing capabilities. It requires a dedicated AI chip to cope with these needs.
For example, in the field of intelligent driving. Deep learning applications such as environmental perception and object recognition require very high computing response speeds. At the same time, power consumption cannot be too high, otherwise, it will affect the car's cruising range. Therefore, developing ASICs is key to industry development.
Will ASIC Replace Other AI Chips in the Future?
ASIC has unique advantages in specific application scenarios, but it also has some limitations.
As its characteristic, an ASIC is designed for a specific algorithm. Once designed, it may no longer be usable if the algorithm changes.
Artificial Intelligence Technology is still in the development stage, and a large number of algorithms are emerging and being continuously optimized. The fixed feature of ASIC prevents it from completely replacing other AI chips to adapt to various algorithms. Although dedicated AI chips can be integrated into SoCs, they can only be accelerated for some specific AI algorithms. For other algorithms, implementation still depends on the CPU and GPU in the SoC.
In addition, cloud servers currently still rely more on CPUs, GPUs, and programmable and reconfigurable FPGAs for artificial intelligence computing and reasoning.
In general, FPGA is more flexible, and under specific algorithms, ASIC is more high-performance and lower cost. As technology develops and algorithms mature and stabilize, optimization of performance and energy efficiency will inevitably be further pursued, and ASICs will also evolve and develop more.